Expert Knowledge and Data Interactions (EDI)
Research Focus:
Analysing clinical observational data benefits from informed constraints put on the modelled relationships of relevant variables also over time because the data are usually heterogeneous and small in terms of the number of available observations.
We are investigating and developing methods to retrieve and operationalise domain knowledge for informed constraining statistical models to extract non-trivial conclusions from biomedical data.
Research encompasses developing domain specific, expert informed, generative models, e.g., for realistic simulations of longitudinal data as in the SmallData project B03 for Epidermolysis bullosa.
Latent embeddings, e.g., as learned with deep generative approaches are considered for denoising potentially high dimensional patient data and to integrate different modalities, e.g., tabular and text data, such as derived from large language models (LLMs).
We also study how LLMs in combination with clinical experts can improve phenomenological models of disease processes.
Preclinical Statistical Analysis Advice:
We provide statistical analysis advice for researchers working with preclinical data such as animal experiments and cell lines, as part of the IMBI statistical consulting service.
- Deep generative approaches
- Unsupervised learning from high dimensional data
- Latent representations
- Mathematical Modelling
- Experimental Design
- Statistical Consulting
- Mathematical Modelling
- Reinforcement Learning
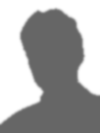
Claire Reiland
- MD Thesis
- Statistical Advice Analysis
- Machine learning
- Mathematical modelling